Terms like Artificial Intelligence or Deep Learning are used generously in today’s conversations, but without explaining their meaning it’s difficult to truly understand the value these technologies bring to businesses. The same is true of Machine Learning (ML), with an increasing number of articles discussing that companies should implement this technology and how it could be beneficial.
However, it goes without saying that businesses and stakeholders should understand Machine Learning and how it can be used in business operations before deciding on its implementation.
In this article, we will explain what Machine Learning is and go through several ways in which it can help you optimise your processes. Let’s dig in!
What is Machine Learning, really?
Machine Learning (ML) has gained a lot of traction in recent years due to its use across a wide variety of industries. From credit card fraud detection to targeted advertising on social media, ML is successfully used for tasks that were once done by humans but can now be automated through algorithms that draw on large databases of information.
Machine Learning is the general term for when computers learn from data. It is a subset of AI where algorithms are used to perform a specific task without being explicitly programmed - instead, they recognize patterns in the data and make predictions based on their learnings once new data arrives.
ML is a great way of automating complex tasks that go further than rule-based automation. Traditionally, developing a Machine Learning solution has been a very costly and time-consuming process. However, thanks to no-code Machine Learning tools like Levity, this technology is now easily available for companies of all sizes.
Three forms of Machine Learning
To understand what Machine Learning is used for in business and how it works, it’s important to know the different ways in which ML can work. Some of the buzzwords you will hear when looking into ML implementation are ‘supervised learning’, ‘unsupervised learning’ and ‘reinforcement learning’ - these are the three most common ways in which machines can learn.
Let’s break down how ML works through a combination of supervised and unsupervised learning
Supervised learning uses data that is already labelled or tagged to train ML models. The algorithms can be trained to correctly categorize data or predict outcomes. As a result, supervised learning lets businesses tackle real-world issues at scale, like separating spam from your email.
Unsupervised learning evaluates and clusters unlabeled data, finding information on its own. These algorithms automatically uncover hidden patterns or data groupings. Compared to supervised learning, unsupervised learning algorithms can handle more complicated problems. Unsupervised learning allows companies to examine data in an exploratory manner, enabling them to discover patterns faster than via human observation.
Supervised learning gathers data from a prior experience or creates a data output from that event. It assists in optimising performance requirements based on previous experience and solving a variety of real-world computing issues.
On the other hand, unsupervised learning finds all kinds of previously undiscovered patterns in data and assists in the discovery of characteristics that are helpful for classification.
Through a mix of supervised and unsupervised learning techniques, a business may classify consumers based on data that is currently available versus data that has yet to be discovered.
One example of how a business may be using both supervised and unsupervised learning is classfiying its customers based on data that is currently available (supervised), versus data that has yet to be discovered (unsupervised).
Another ML technique is reinforcement learning. Reinforcement learning trains computer models to make choices by placing the AI in a game-like situation.
In reinforcement learning, the algorithm learns through trial and error using feedback from its actions. Rewards and punishment operate as signals for desired and undesired behaviour.
Through rewards and punishment, the ML model gets positive and negative feedback on its behaviour and learns from its own experience to maximise the accuracy of its output.
What are some applications of Machine Learning in business?
Machine Learning can be used in many areas to optimise your processes and workflows, from marketing and advertising, to customer support and product research. Here are some of its top applications.
Sorting and routing incoming emails
Use an AI-powered tool to automate email sorting into different actionable datasets. You can opt to respond manually, automatically, or be alerted of urgent requests based on the tag.
For example, if the email response is categorized as Out-Of-Office, you can send another reminder to this prospect after a week.
You may also receive specific insights on the performance of your campaign by aggregating the categorized answers in one place. You can then run analytics on your data to uncover greater details by integrating your model with other solutions.
Here you can read how Levity helps a lead generation agency automate sorting email campaign responses and save hours of manual work.

Content moderation and generation
Content moderation is a popular example of a time-consuming, and error-prone activity. Automation is the only way to get this right, and you don’t need to look further than ML automation to make moderation easy.
Levity's content moderation feature lets you quickly search through thousands of images and a large amount of text, freeing up your team's time to focus on more critical tasks.
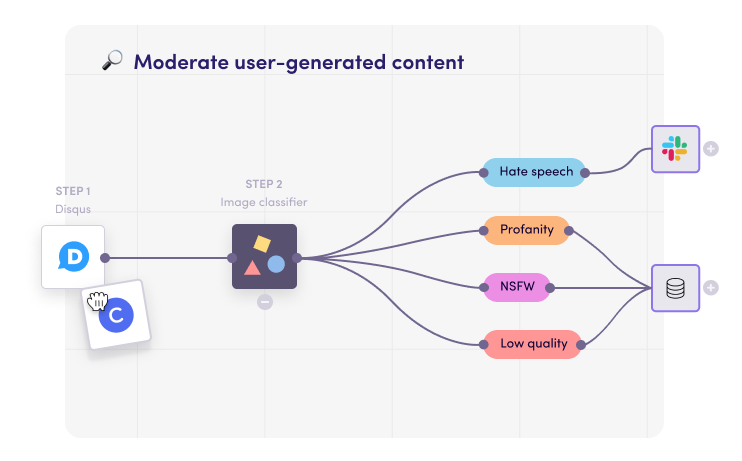
Tag email attachments
Tagging email attachments can be troublesome and tedious. Document classification categorizes emails by attachment type, such as PDFs, images, or spreadsheets. It further routes emails with attachments to the appropriate team or department.
This ensures documents get to the right department the first time around. Finance doesn’t want to be sending HR contracts about as much as HR doesn’t want to spend their time forwarding invoices to finance.
With Levity’s email categorization workflows, you can ensure your team is able to focus on their tasks and their tasks alone.
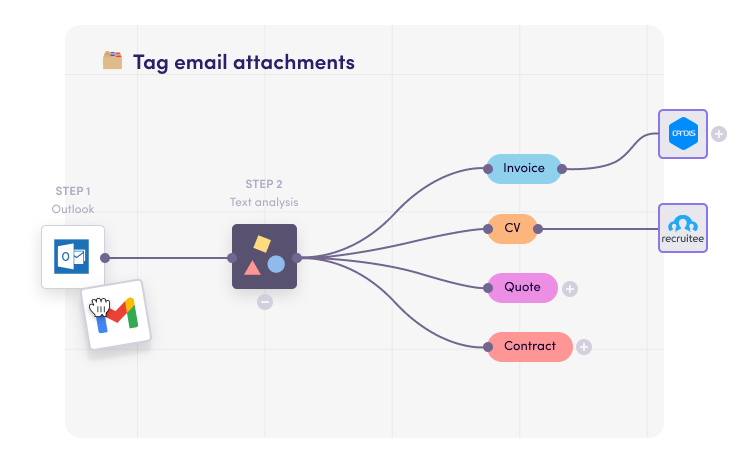