Your customers have emotions and feelings.
Recognizing, addressing, and appreciating that your customers associate emotional value with your brand, product, or service helps you address those feelings—be they negative or positive.
Understanding what customers say or feel about your brand is critical to creating a positive customer experience and brand image.
Let's say you want to monitor your content for different tones—such as sad, happy, frustrated, and joyful—flag inappropriate or negative comments, or analyze search results. You’re eager to learn how customers interact with different facets of your business and their thoughts and opinions around them.
But…
You don't have the time and resources to analyze all of this data manually, or just don't want to spend your valuable time—that could be better spent elsewhere—manually classifying sentiment.
How do you ensure you’re able to manage the many moving parts of your business while successfully understanding your customers’ thoughts and feelings? Is what you’re doing worth it, or should you try something else?
The sweet and short answer: sentiment classification.
Sentiment classification helps you answer questions like:
- What are customers saying about your brand?
- Are they satisfied or dissatisfied with your services?
- What do they like or dislike about the user experience?
- What can you do better to address their concerns and issues?
When you make an effort to know what your customers feel, you stand a better chance of attracting and retaining them. Sentiment classification is a powerful technique to help you do just that.
Let’s start with the basics.
What is sentiment classification?
Sentiment classification is the automated process of identifying and classifying emotions in text as positive sentiment, negative sentiment, or neutral sentiment based on the opinions expressed within. It helps determine the nature and extent of feelings conveyed using Natural Language Processing (NLP) to understand what customers say or feel about your brand, products, and services.
Manually reading, classifying, and sorting text data can take a long time. You can lose the essence of what's important when you get stuck manually analyzing and classifying positive and negative sentiments.
Sentiment classification has seen tremendous growth in recent years. Many businesses use it to better understand their customers' feelings, behavior, preferences, and needs.
Automated classification methods enable you to analyze reviews, comments, survey responses, and other public opinions faster. They analyze data to determine the overall tone toward your brand and products, as well as the broader industry and market trends and insights.
Sentiment classification applications
Sentiment classification can be used in many areas, from marketing and advertising to customer service and product research. Here are some of its top applications.
Customer service
Customer service teams can use sentiment classification to understand customers better. Using NLP and sentiment analysis-based tools, they can identify, prioritize, and resolve customer issues faster.
When analyzing customer feedback, you can use sentiment classification to spot different trends. By monitoring feedback sentiment, you can uncover patterns that indicate if your team meets or exceeds expectations and identify areas for improvement. This allows you to focus on the specific areas that matter most to your customers.
The easiest way to do sentiment classification is with Artificial Intelligence (AI) tools. Automation accelerates your processes and makes them more efficient.
A no-code AI tool like Levity simplifies classifying sentiment in text data. You also get multiple integrations with platforms like Slack and Zapier to pull your data and automatically notify relevant people based on the sentiments classified from customer data.
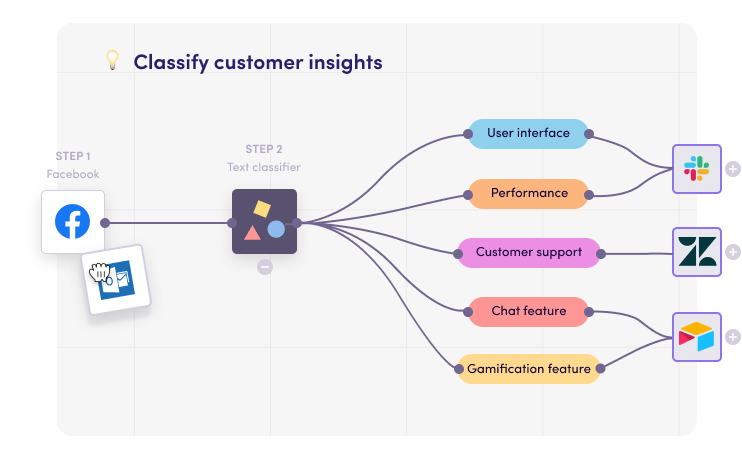
Social media monitoring
Social media is crucial for gaining insights into what people think about a given topic—in this case, your brand.
However, when it comes to social media monitoring and social listening, it's easy to get overwhelmed by the sheer quantity of feedback and data to monitor. Automated sentiment classification is a winner when it comes to monitoring, understanding, and responding to social media conversations and addressing complaints and concerns.
With sentiment classification, you can easily monitor social media platforms and see which topics are getting the most attention—whether that’s positive or negative. Social media sentiment classification is a great way to stay ahead of emerging trends and capture negative reviews early on.
This allows your team to proactively work toward a solution and nurture a positive customer experience in the future.
Practicing social listening is the first step in helping you understand the sentiment behind a social review, post, or comment. With an integrated AI platform like Levity, you can easily hear what people talk about you, track keyword engagement, cut through the noise, and prioritize insights—all without lifting a finger.

Market and competitive research
Since most business is done online, the competition has reached another level. Market and competitive research are both essential for monitoring sales growth and product success.
Keeping track of your competitors or key market trends can be hectic and time-consuming. Sentiment classification tools save the day when it comes to understanding the market, identifying potential competitors, and discovering customer trends and purchasing habits.
The ability to quickly gauge sentiment is key to any market research exercise. Therefore, it’s essential to use the right tool to classify sentiment correctly and get quick insights. By closely checking sentiment around your brand, you can prioritize customer issues, segment customers, and improve customer satisfaction with appropriate solutions.
