Resources
Automation strategies, ideas and practical guides.

Customer Success Story: How FRAYT Scaled Smarter with Levity’s AI Workflows
In the logistics industry, speed wins. For Frayt, a rapidly growing last-mile delivery platform, success depends on responding quickly, quoting accurately, and scaling without sacrificing quality.
May 5, 2025

Outsmarting the Giants: AI in Freight Brokerage
The freight brokerage industry is evolving fast, but smaller brokers can still stay competitive by using AI to boost efficiency and reduce costs.
Sep 30, 2024

Customer Success Story: How Project Apollo is transforming freight brokerage email operations with Levity
Project Apollo sought a partner capable of revolutionizing their email-based communication to carriers with a focus on quoting and tendering freight.
May 5, 2024
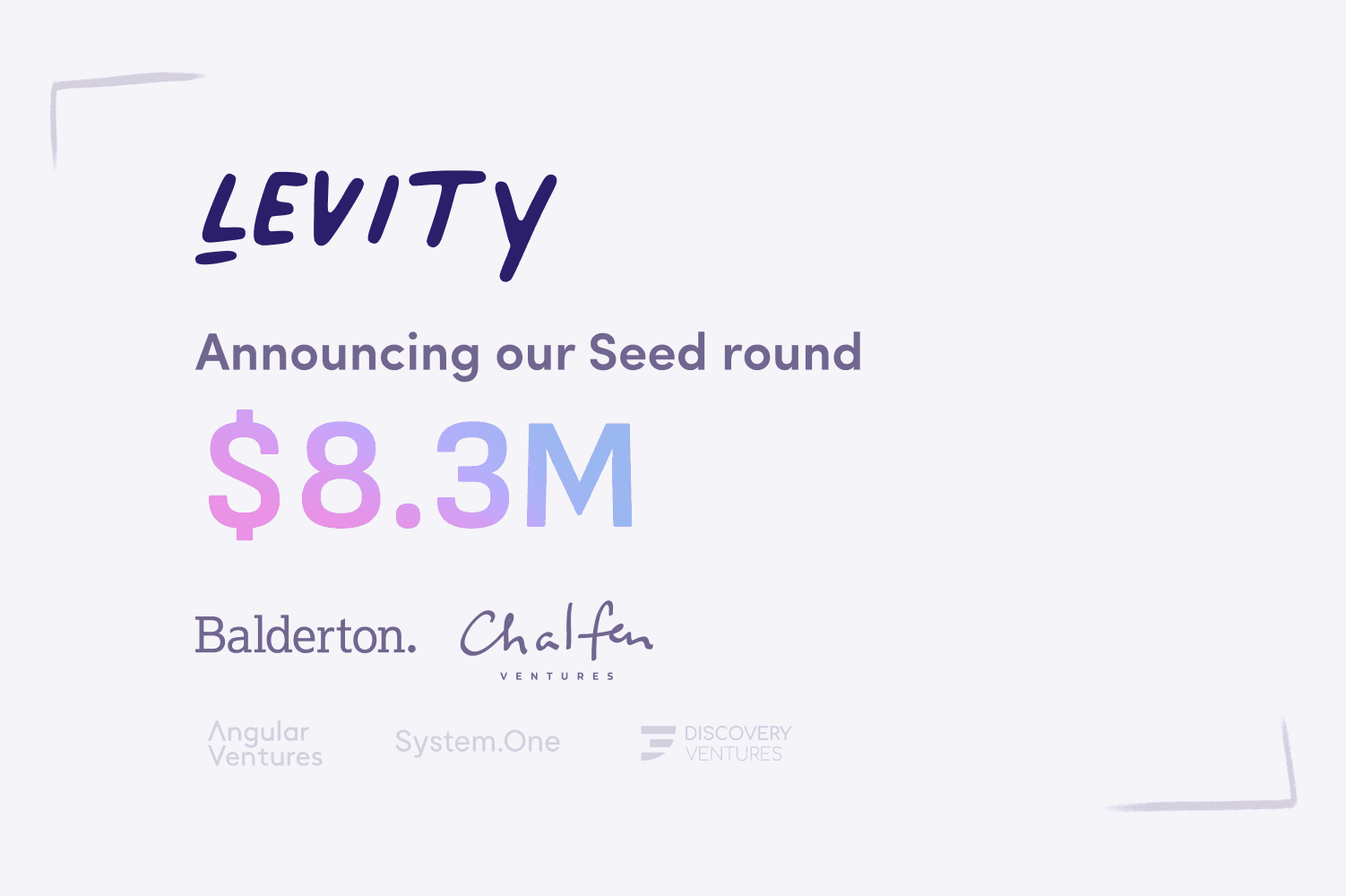
Announcing our Seed Round
We're beyond excited to announce that we've raised an $8.3M Seed round co-led by Balderton Capital and Chalfen Ventures.
Oct 12, 2022