Identifying insights within your company’s data is no easy task.
Most companies use data analytics tools to make business decisions, but these are often limited to structured data, such as spreadsheets or SVG files. If you are not pulling insights from your unstructured data - emails, comments, documents, images, etc.- you are missing out on key insights, as most data generated by businesses falls under this category.
But with next-generation data analysis tools, you can immediately start uncovering insights that were hidden under large volumes of unstructured data.
In this article, we’re going to explain the differences between structured, unstructured, and semi-structured data, and show you how each of these is used in AI tools to provide value for your business.

Structured, unstructured, and semi-structured data
Let’s start off by clarifying how unstructured data differs from structured data.
What is structured data?
Structured data is organized, easy-to-understand information that follows a set data model. It's often stored in databases like Excel files or SQL so it can be accessed and understood by both humans and programs.
Even though it only comprises around 20% of the data worldwide, it is the foundation of the widely known ‘Big Data’.
Structured data examples
CRM (customer relationship management) systems store customer information such as name, address, email, mobile number, and date of birth - all examples of structured data. Each of these pieces of data is formatted in an organized and consistent way from one customer to another meaning the systems are designed to work with predetermined information structure.
Standard systems used for CRM, inventory control, order management, and others, also handle structured datasets.

The purpose of a database, such as this table structure, is to store information in an organized way so that it can be easily accessed, updated, or deleted. These databases also offer a domain-specific language – the famous SQL - that facilitates data manipulation.
What is unstructured data?
In contrast to structured data, unstructured data is information that doesn’t have a predetermined data model and can’t be stored in a traditional relational database.
Long text, images, and videos are generally categorized as unstructured data. Emails, social media content, chat messages, and web forum content are some of the most common sources of unstructured data today’s companies have to deal with. Business documents like legal contracts and customer survey questionnaires contain huge amounts of this type of data.
Unfortunately, because it doesn't follow a designated structure, unstructured data can be hard to analyze if tackled manually. This often leads businesses to simply ignore this type of data, missing out on valuable insights.
However, thanks to the development of AI-powered tools like Levity, it is now possible to classify unstructured data faster and more efficiently than ever before. These tools are redefining the way we process this type of data and allow us to get the most out of unorganized data with minimal effort.
Unstructured data examples
Let’s look at two unstructured data examples.
A standard email has a sender, one or more receivers, sent time, and a message body, Sometimes, it includes one or more attachments as well. The senders, receivers, and time sent, fit into a structured data model but the message body contains unstructured information. If we don't read the entire email, it's impossible to determine its meaning and context.
Social media content is another example of both structured and unstructured data. Some information such as username and time of activity is structured data, but analyzing this information will give limited actionable insights. To gain a real understanding, we have to tap into the content which includes free-form text, pictures, and often videos. This type of information is inherently unstructured and does not follow a structured data model.
Unlike structured data, unstructured data cannot be arranged into tables with fixed columns and rows quite so neatly. It is generally stored in so-called ‘data lakes’ as raw information in their native format.
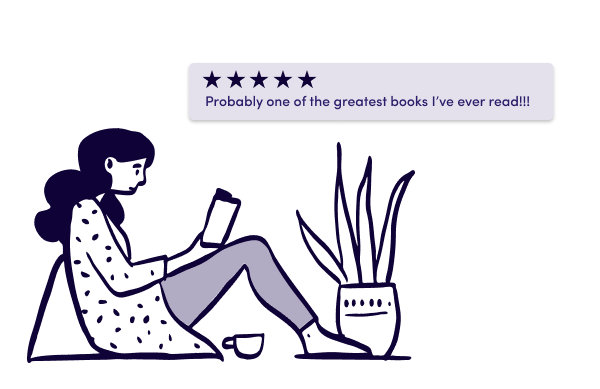
Structured vs unstructured data in next-gen tools
Classifying unstructured data manually is a time-and-resource-consuming activity. Traditional data analytics tools are not capable of handling the volume and complexity of this data and businesses were left with no scalable solutions
next-generation data analytics tools are changing the game on this front. Built on AI and ML, these tools can analyze unstructured data and provide valuable insights. One such tool is Levity. Let’s take a closer look.