Often described as the silver bullet for all of humanity’s woes, Artificial Intelligence is seen both as the next game-changing innovation by some and also as the technology that will bring about the end of human society by others. But the truth, however, is somewhere in the middle and also muddled for the most part.
AI is merely a collection of techniques to aid a computer’s perception of information. It is for all intents and purposes, a multi-purpose tool. A tool whose true extent of abilities and uses are largely speculative.
As Michael Jordan, a professor in the Department of Electrical Engineering and Computer Sciences at UC Berkeley suggests in his article, this lack of definition has led AI to be applied in so many diverse use cases that it has practically lost all meaning. AI is in everything and everywhere and yet means nothing to most people.
Perception of AI and the role of UX
AI is rapidly permeating every industry and product category as engineers attempt to tap into its true potential, but the question remains, how do users perceive AI amid such ambiguity around its purpose?
Users’ perception is undoubtedly an integral part of any new technology’s acceptance and widespread adoption in society. The promises which AI comes with serve as a compelling argument as to why it deserves widespread adoption in our society. But some studies indicate that people have a “mixed perception” of AI, with its popular perception often leaning towards utopian or dystopian extremes; and alarmist narratives like these could affect its adoption.
This is where design comes in.
Good UX has often served as the step-ladder when getting users to come on board with whatever wild new innovations the engineers have conjured up - be it Apple’s iPhone, which convinced people to carry around a fragile glass computer in their pockets; or Amazon’s Alexa, which normalized people shouting commands to an internet-connected microphone in their homes.
Neither the iPhone nor the Alexa kickstarted new product categories but both products’ unique selling proposition was a drastic improvement in the user experience (UX) department. This was what enabled them to foster widespread adoption in their respective product categories.
However, developing well-rounded user experiences for AI products comes with a lot of questions and challenges. Challenges around how to provide the most relevant information to help users understand the AI’s value and decisions while not flustering them with complexity.
Questions around how to better design AI products - how to provide relevant information to help users understand their value and decisions - are considered to be one of the critical and pervasive design issues for AI systems.
Tackling these UX challenges would serve many user needs such as improving, contesting, developing appropriate trust, better interacting with AI, and eventually accomplishing the user goal. Although currently, explainability features are still not a common presence in consumer AI products.
Designing for AI is not entirely uncharted territory for designers. Twitter, TikTok, Snapchat, and even streaming services such as Spotify and Netflix are good examples of when AI-driven features/functionality were successfully integrated into a mass-market product with little controversy.
There seem to be two different approaches to this:
1. The Black-Box Approach
In the pursuit of improving the experience and maintaining ease of use, designers intentionally obscure the AI-powered parts of the product irrelevant to the users’ goals. The designers here take the “black-box” approach. As the name suggests, in this approach designers avoid referring to AI or other AI-related terminology in an attempt to keep things simple.
A notable example of this is Spotify and its “Discover Weekly” playlist.
Every Monday, over 180 million Spotify users are presented with a new playlist, custom-made to their unique taste in music. To the user, it looks just like any other playlist, but under the hood, this is essentially a playlist made using AI. A Machine Learning model learns the users’ listening patterns and processes this data to make this playlist. In essence, when the user listens, skips, likes, and searches for songs, they are actually training an ML model without knowing it.
It is easy to see why the designers at Spotify took this approach; it just doesn’t make a whole lot of sense to explain Convolutional Neural Networks to a user who is just looking for some music to play on their way to work.

Twitter, also employs a similar approach, ranking tweets based on relevance to its users, the number of times it has been re-tweeted, potential hate speech, etc.
Although the approach is similar, there is a key difference here: Twitter’s AI is not sorting songs, its sorting opinions, and ideas. This has huge consequences since it could influence the users’ personal opinions.
While there’s an AI model with potential bias sorting out tweets for the user feed, the user unaware of this is consuming this feed of ideas; raising questions about free speech, propaganda, feedback loops, and censorship.
Another case where the black-boxing approach caused issues was in 2019 when several users including Apple’s co-founder Steve Wozniak encountered gender bias in Apple Card’s AI model. In Wozniak’s case, the AI automatically set a credit limit for his wife which was ten times lower than his, despite the fact that they both shared the same assets; all without providing a clear explanation.
This shows us that the need for explainability is extremely important in AI systems that are responsible for influencing users’ decisions and actions. The major fault of this approach is that it compromises explainability in pursuit of keeping things simple. This line of thought is where the Transparent approach comes from.
2. The Transparent Approach
In this approach, designers utilize a combination of simple text and UI elements so that even users with limited technical knowledge can readily understand the AI’s role in the product.
Explanations are crucial for building user trust, but due to the complex nature of the domain, offering explanations of an AI’s functionality can be a challenge in and of itself.
Despite its name, the idea is not to explain everything, but just the aspects of the product that impact the users’ trust and influences their decision-making.
Notable examples include SaaS products such as Grammarly and Wix or even Apple’s Photos app which take this approach of being transparent about the AI-powered nature of their app’s features.
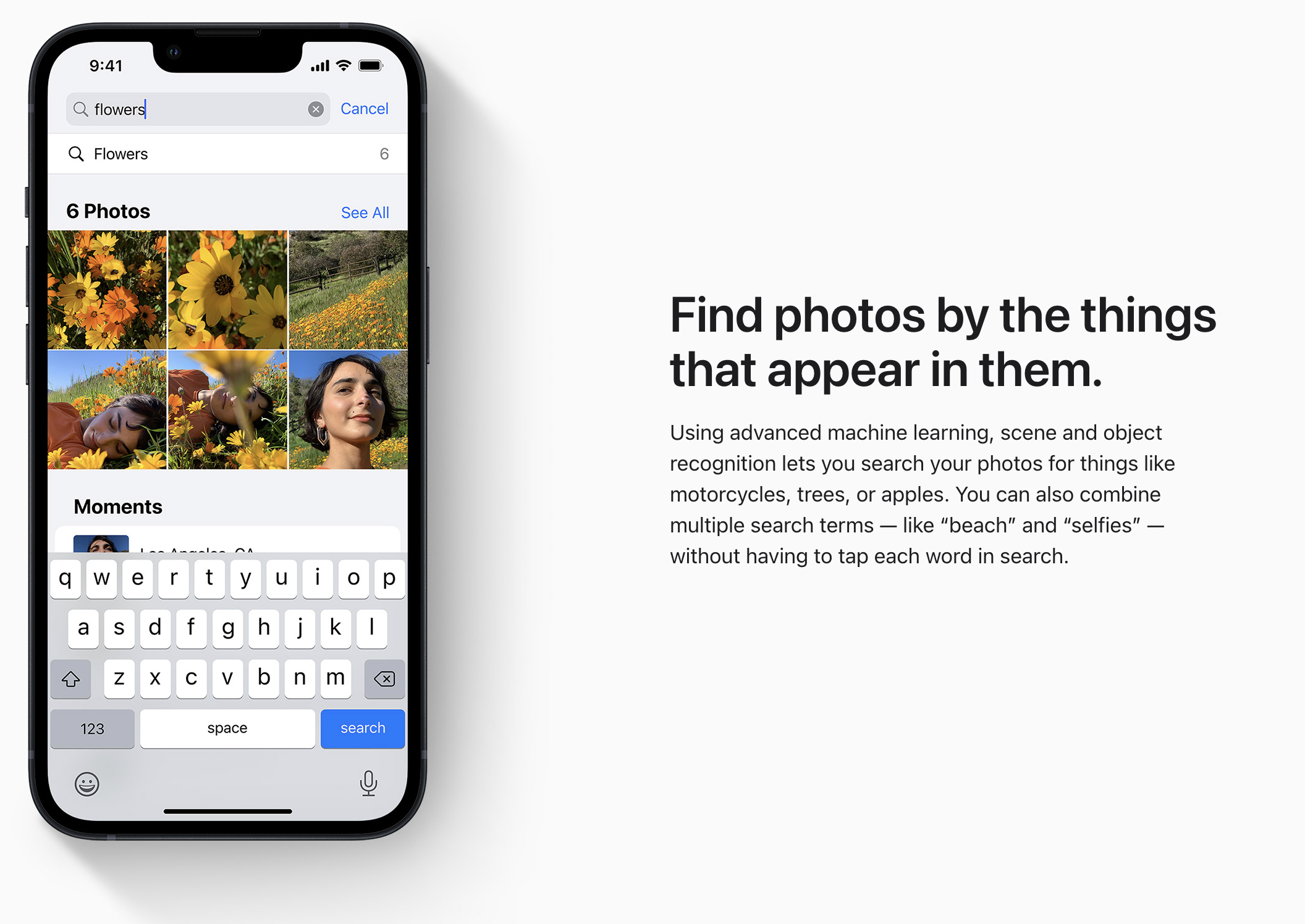
Users “seek explanations so that they can build their trust and confidence in an AI system’s recommendations”. While a quick fix for this would be providing information about the AI system in use, it does not solve the problem for the majority of users who are not familiar with AI or concepts in the Machine Learning field.- UXAI
Non-technical users may also have complex use cases, but will also have less tolerance for complex explanations. Therefore, finding the right balance between these approaches is key to achieving successful user journeys in AI-powered products.
Finding the right balance and other considerations
Irrespective of the approach adopted, when designing for AI, the designer is met with a plethora of challenges, such as explainability, mechanisms of user feedback, etc. But one of the major challenges when designing for AI is to strike the right balance between transparency and ease of use.
Now, let’s break down the key considerations and challenges when designing an intuitive but transparent AI:
1. Managing expectations
Predictions not truths
AI may be portrayed in much of the media as our new overlord, dictating policy while casually making restaurant reservations for us, but these systems are, dare I say actually quite humble.
In AI-driven products, more often than not, AI predictions are presented to the users as certain truths, rather than probable outcomes. This results in either the AI being perceived as too intelligent for its own good or too basic and “simple-minded” to be relied upon.
Take, for example, an AI model that has learned to identify faces labeled as “smiling”. Behind the scenes, the model is probably looking for a horizontal set of light pixels (which we humans would call “teeth”) in the bottom third of the image. This means that the AI doesn’t know anything about smiling or the human emotion of joy, it only thinks that there’s say, a 71.23% chance that this might be a smiling face. AI models only yield probabilistic guesses, not judgments.
All predictions come with confidence scores that indicate how sure the AI is about something. Sometimes, the confidence isn’t high enough and there shouldn’t be any shame in admitting that. Like the AI, we too aren’t 100% sure/right about all things, all the time. We too, like the AI we are building only have degrees of confidence.
Mental models
Di Dang, the co-creator of Google’s People + AI Guidebook suggests using mental models as a way to help set expectations when designing AI products. Mental models are guides that our brains put together to help us figure out how something works and how our actions affect it. We all have mental models for everything we interact with - products, places, and even people.
In the case of products, they help set expectations for what a product can and can’t do and what kind of value people can expect to get from it. Incorrect mental models can lead to user friction and frustration.
Due to their nature, mental models can’t be explicitly designed, but they can be designed for:
- Identifying existing mental models.
The easiest way to leverage mental models is to tap into the users’ existing ones. This ensures that the AI in the product already follows a similar process pattern to the user in solving the same problem or accomplishing that goal.
- Adjusting and recalibrating mental models for responsive products.
Take the example of a physical mixtape. A mixtape made in the 90s is going to be the same tomorrow. Now, contrast that with a modern streaming service that dynamically adjusts its recommendations based on user interactions, like Spotify. AI-powered products respond to user interaction to get better over time and as a result, the user experience can change. Designers can prime the users for this, by adding “inboarding” messages allowing the users to adjust their mental model as necessary.
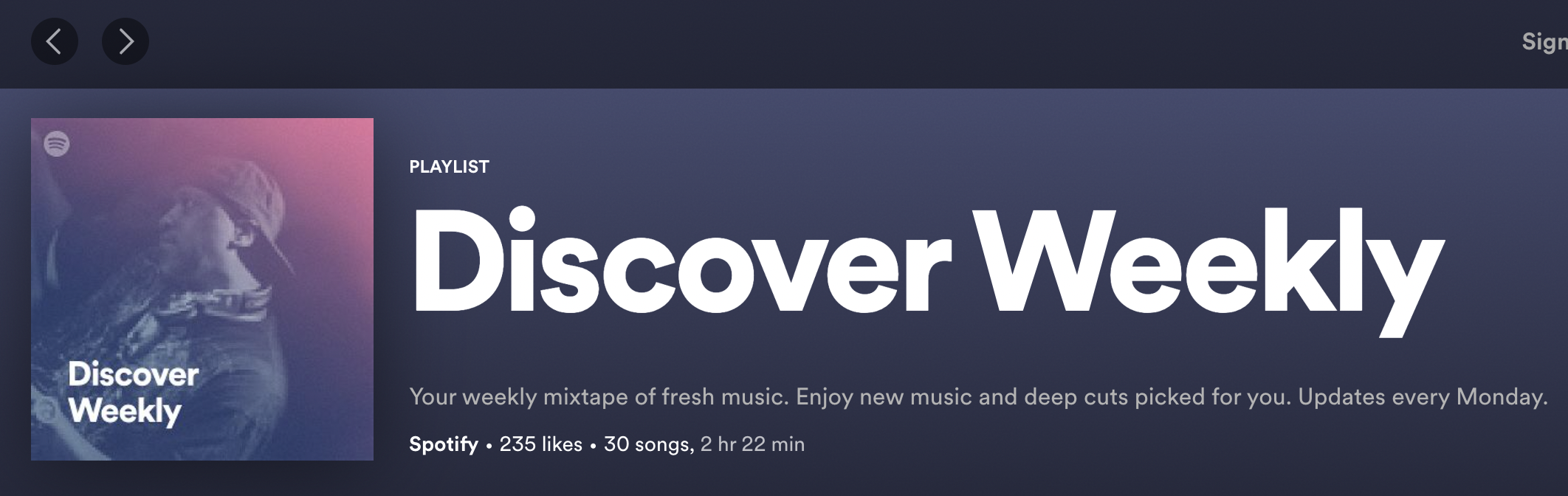
When designers invest in communicating within their products that an AI’s predictions are just that, predictions, not only does it set the right expectations it also makes for a more transparent experience sans unmet expectations, frustration, and product abandonment.
2. Calibrating trust
AI-driven products often set their users up for failure by promising them that some “AI magic” will help them accomplish their goals. In more complex use cases, this can establish a misplaced trust that drives users to overestimate the product’s abilities. While the “black-box” approach aims to simplify the UX, completely obscuring the “how” can confuse users, break the experience, and even erode the users’ trust.
Therefore it becomes necessary to introduce and present the product’s AI features in a way that sets realistic expectations to build a level of trust that is calibrated to the product’s real-world capabilities.
Progressive disclosure
One of the ways to build the right level of trust with users is by explaining features right when the user might find a need for them (i.e. in the moment). As users begin interacting with the product, actionable “inboarding” messages can be used to briefly convey relevant information to help them along. This is known as progressive disclosure.
Progressive disclosure is a practice in UX when additional information is revealed progressively in subsequent screens or interactions.
While this is usually done to keep the onboarding journey brief, it can also be employed to avoid introducing new concepts when users are occupied with another unrelated task.
As discussed earlier, AI-powered products respond to user interaction to get better over time resulting in changes to the function or user experience. Calibrated trust can be achieved by teaching the users in short, explicit bites of information right when they need it.
Focusing on the value, not the technology
As highlighted earlier, people have a “mixed perception” of AI and because of misconceptions about AI, users either trust it too much or too little. When a product mentions that it employs AI to solve a problem, users may wonder what the product can and can’t do, how it works, and how they should interact with it. While it is almost impossible for a product to clarify all the misconceptions a user may have about the underlying technology, there is a lot of value in focusing on the value that the product offers.
- Using the onboarding journey to communicate the product’s capabilities and limitations clearly, set expectations early on.
- Start out with the users in control, and introduce automation gradually under the users’ supervision. Allowing users to get used to the AI taking on smaller tasks while making sure that they can provide feedback to guide this process. This demonstrates value to the user while still being in control.
Highlighting the AI’s usefulness and being realistic about its’ abilities is one of the ways, calibrated trust can be built.
Levity’s take on UX for AI
At Levity, when designing the app, we try to incorporate transparency in areas such as labeling data and training an AI block but in the same vein, we black-box some of the more technical aspects of building an AI model - such as training data passes, data transformation instructions, regularization type, etc. This is done to distill the process of building and training the ML model (which we refer to as an AI Block) as straightforward and intuitively as possible.
While there are a lot more features that we plan to incorporate into the app based on the capabilities of the AI we use, we try to first focus on finding that balance between explainability and complexity. Because that’s key to our product vision and we’d like to add some levity to a user’s experience with AI.

To sum up...
The role of UX in AI products is less about highlighting the underlying technology and more about providing users with a better, clearer overview of the value that AI affords. This is key as AI becomes ever more present and influential in our daily lives.
For some products like Spotify, the solution to this challenge is quite an easy one - black-box the AI features to keep things simple and relevant to the app’s main objectives. However, for applications with more complex uses of AI, (such as social media or no-code platforms) the solution to this challenge is no longer an easy one. As Nadia Piet states in her article, when designing for such AI, it is the duty of designers to “help them (the users) understand how they work, be transparent about their abilities, construct helpful mental models, and make the users feel comfortable in their interactions”.
Why is this so critical? As discussed earlier, a sizable majority of people still don’t trust AI, either for its abilities or for its virtues; and no technology will ever reach its true potential until it earns the users’ trust. That’s why this is important.
Ideally, we’d want something that serves the users’ goals without either making them feel like a 5-year-old or like they need a Ph.D. to even begin using this thing. The solution is neither full transparency nor black boxing, the solution lies in finding that balance between transparency and black boxing. Once we’ve found the right opacity between these two approaches, we may have just designed something that users trust, confidently use, and hopefully even find some delight in.